Artificial Intelligence (AI) has revolutionized various industries, and one of the most impactful applications is AI-based image recognition software. This technology enables computers to identify and process images much like the human brain, with a level of accuracy and speed that is continually improving. From facial recognition and autonomous vehicles to healthcare diagnostics and retail, AI-powered image recognition is transforming industries and reshaping how we interact with technology.
In this article, we’ll explore how AI-based image recognition software works, the key technologies behind it, its wide range of applications, and its impact on industries and society at large.
What is AI-Based Image Recognition?
AI-based image recognition refers to the ability of computers to identify objects, people, places, and other items in digital images or videos. Using machine learning (ML) and deep learning algorithms, AI systems are trained to recognize patterns and features within images, enabling them to label objects or detect differences with remarkable precision.
This technology goes beyond traditional computer vision, as AI systems can learn from vast amounts of visual data, continuously improving their ability to recognize more complex images. One of the most well-known examples of this technology in everyday life is facial recognition, which identifies and verifies a person’s identity based on facial features.
How Does AI-Based Image Recognition Work?
AI-based image recognition relies on deep learning models, specifically convolutional neural networks (CNNs). CNNs are designed to simulate the way the human brain processes visual information by analyzing images in layers, detecting edges, shapes, textures, and patterns, and then recognizing these elements to make sense of the overall image.
Here’s a step-by-step breakdown of how AI-based image recognition works:
1. Data Collection and Labeling
The first step in developing an image recognition system is to gather a large dataset of labeled images. For instance, if the goal is to create an AI model that recognizes dogs, thousands of images of dogs (labeled as such) must be collected and used to train the model.
2. Training the AI Model
Using machine learning techniques, the system is trained on the dataset. This involves feeding the images into a deep learning model (typically a convolutional neural network) so that the model can learn to identify features, such as shapes, colors, and textures. Over time, the system begins to understand what a “dog” looks like by identifying specific patterns associated with dogs.
3. Feature Extraction
The AI model processes the images through several layers, each extracting different features from the image. The early layers might detect edges, while later layers identify more complex features like eyes, ears, or fur texture.
4. Classification
Once the features are extracted, the model makes predictions about what the image represents. In the dog example, the AI might compare the image to what it has learned and classify it as a “dog” if it matches the patterns it has previously learned.
5. Feedback and Improvement
AI-based image recognition systems are designed to improve over time. By receiving feedback—either from human input or from further analysis of new images—the system refines its algorithms, increasing its accuracy and reducing errors.
Key Technologies Behind AI Image Recognition
Several key technologies enable AI-based image recognition software to function effectively:
1. Convolutional Neural Networks (CNNs)
CNNs are the backbone of modern image recognition systems. Unlike traditional neural networks, CNNs are specifically designed to process pixel data in images. They consist of multiple layers, each performing different functions, such as filtering, pooling, and rectification. These processes allow CNNs to recognize specific features and patterns within an image and classify them accurately.
2. Deep Learning
Deep learning is a subset of machine learning that uses large neural networks to simulate the human brain’s learning process. In image recognition, deep learning models (like CNNs) can process and analyze massive amounts of visual data. These models are “trained” on vast datasets, learning from the images they analyze and improving their ability to make accurate predictions over time.
3. Artificial Neural Networks (ANNs)
Artificial neural networks are the basis for both CNNs and deep learning. These networks are designed to mimic the neurons in the human brain, with layers of interconnected “neurons” that process data. In image recognition, ANNs allow systems to detect patterns and relationships between pixels, facilitating the identification of objects or people.
4. Natural Language Processing (NLP)
Although not directly related to image recognition, NLP can be integrated into AI systems to improve understanding and contextualization. For example, AI systems might use NLP to “read” text from images, such as street signs or product labels, and provide relevant information or instructions.
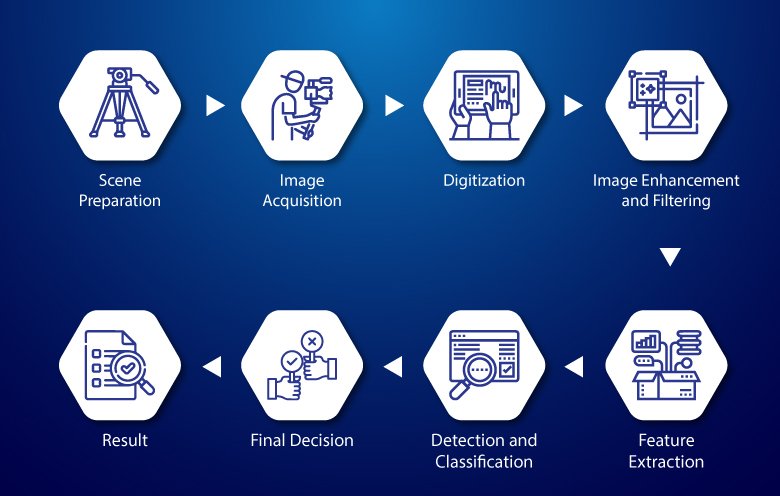
Applications of AI-Based Image Recognition
AI-based image recognition is used in numerous industries and has a wide range of practical applications. Here are some of the most notable ones:
1. Facial Recognition
One of the most widespread uses of AI-based image recognition is facial recognition technology. It’s used in various sectors, including security, law enforcement, and even in consumer devices like smartphones. AI-powered facial recognition systems can identify individuals by analyzing facial features and comparing them to a database, allowing for verification and authentication.
2. Healthcare and Medical Imaging
In the healthcare sector, AI-based image recognition is transforming diagnostics. Medical professionals use AI to analyze medical images, such as X-rays, CT scans, and MRIs, to detect abnormalities and diseases like cancer. AI systems can assist doctors by highlighting areas of concern and improving diagnostic accuracy, ultimately leading to faster and more effective treatments.
3. Autonomous Vehicles
AI-based image recognition is essential for the development of autonomous vehicles. Self-driving cars rely on AI to recognize and interpret their surroundings, including detecting objects, pedestrians, traffic signs, and road conditions. These systems help vehicles make real-time decisions, improving safety and navigation.
4. Retail and E-Commerce
In retail, AI-powered image recognition is used for product identification, inventory management, and personalized recommendations. For instance, retailers can use AI to recognize products in photos uploaded by customers and suggest similar items for purchase. In e-commerce, image recognition allows customers to search for products using images, making shopping more intuitive and efficient.
5. Security and Surveillance
AI-based image recognition is widely used in security and surveillance systems to identify suspicious behavior, track individuals, and monitor locations in real-time. AI can analyze footage from security cameras and alert operators when it detects unusual activity or specific people of interest, enhancing public safety and security.
6. Agriculture
Farmers use AI image recognition to monitor crops, identify plant diseases, and assess soil health. By analyzing images captured by drones or satellites, AI systems can detect early signs of disease or pest infestations, enabling farmers to take timely action to protect their crops and improve yields.
Challenges and Ethical Considerations
While AI-based image recognition offers numerous benefits, it also raises several challenges and ethical concerns. Some of the key challenges include:
1. Privacy Concerns
Facial recognition and surveillance technologies have raised significant privacy issues. The use of AI to monitor individuals without their consent can lead to misuse, particularly in regions with limited regulations. Companies and governments must address these concerns and ensure that AI is used responsibly and transparently.
2. Bias and Discrimination
AI systems are only as good as the data they are trained on. If the training data is biased or unrepresentative, AI models can make biased decisions. For example, facial recognition systems have been criticized for having higher error rates when identifying people of color, leading to concerns about discrimination and inequality.
3. Regulation and Accountability
As AI-based image recognition becomes more widespread, there is a growing need for regulation to ensure its ethical use. Governments and organizations must establish guidelines and policies to govern the deployment of AI, ensuring that it is used in ways that protect individuals’ rights and freedoms.
The Future of AI-Based Image Recognition
The future of AI-based image recognition is incredibly promising. With advancements in deep learning, computing power, and access to large datasets, image recognition systems will continue to become more accurate, efficient, and versatile. As these systems evolve, we can expect to see even broader adoption across industries, from healthcare and security to entertainment and education.
Emerging technologies like quantum computing and edge AI will further enhance the capabilities of image recognition systems, enabling real-time analysis and decision-making in more complex scenarios. Additionally, as ethical frameworks and regulations catch up with technological advancements, AI-based image recognition will become more widely accepted and integrated into everyday life.
Conclusion
AI-based image recognition software is revolutionizing the way we interact with the digital and physical worlds. From healthcare diagnostics and autonomous vehicles to security systems and retail applications, this technology is reshaping industries and unlocking new possibilities. While challenges remain, particularly around privacy and bias, the potential benefits of AI-powered image recognition are vast and far-reaching, making it one of the most exciting and transformative technologies of our time.